The Need for Better Data: Why Data Quality is Essential
This is Part 3 in our series on Operational Data Platforms. Today we focus on Data Quality in OT. When is data ‘good’? And why is it essential in your IT/OT Convergence journey?
Last year we published two articles about the Operational Data Platform. In Part 1 we discussed ways to handle (sensor) data and the differences with IT. In Part 2 we dived into the importance of looking at your data from different viewpoints and as such introduced the need for working with qualitative data and having a way to manage that data.
In this article we focus on Data Quality.
🤔When is data ‘good’?
🤔Why does that matter for IT and OT?
🤔Why is it even more important for users consuming your data products?
The Perfect World: From Data to Business-Ready-Info
Here is how we rely on data today:
Sensors (either traditional or IIoT) capture data which gets stored in an historian somewhere.
For those in Stage 1 of the Data Maturity Model: this is all you have available as users directly access your historian. Those in Stage 2 or further can take additional steps.
Sensor data gets augmented in the IT/OT zone, for example by MES systems including Asset and/or Process Context (‘what was I producing’, ‘where’ and ‘when’).
Finally, enhanced and transformed data is made available as business ready information to the end user.
Sounds pretty straightforward, but lots of things can (and do) go wrong.
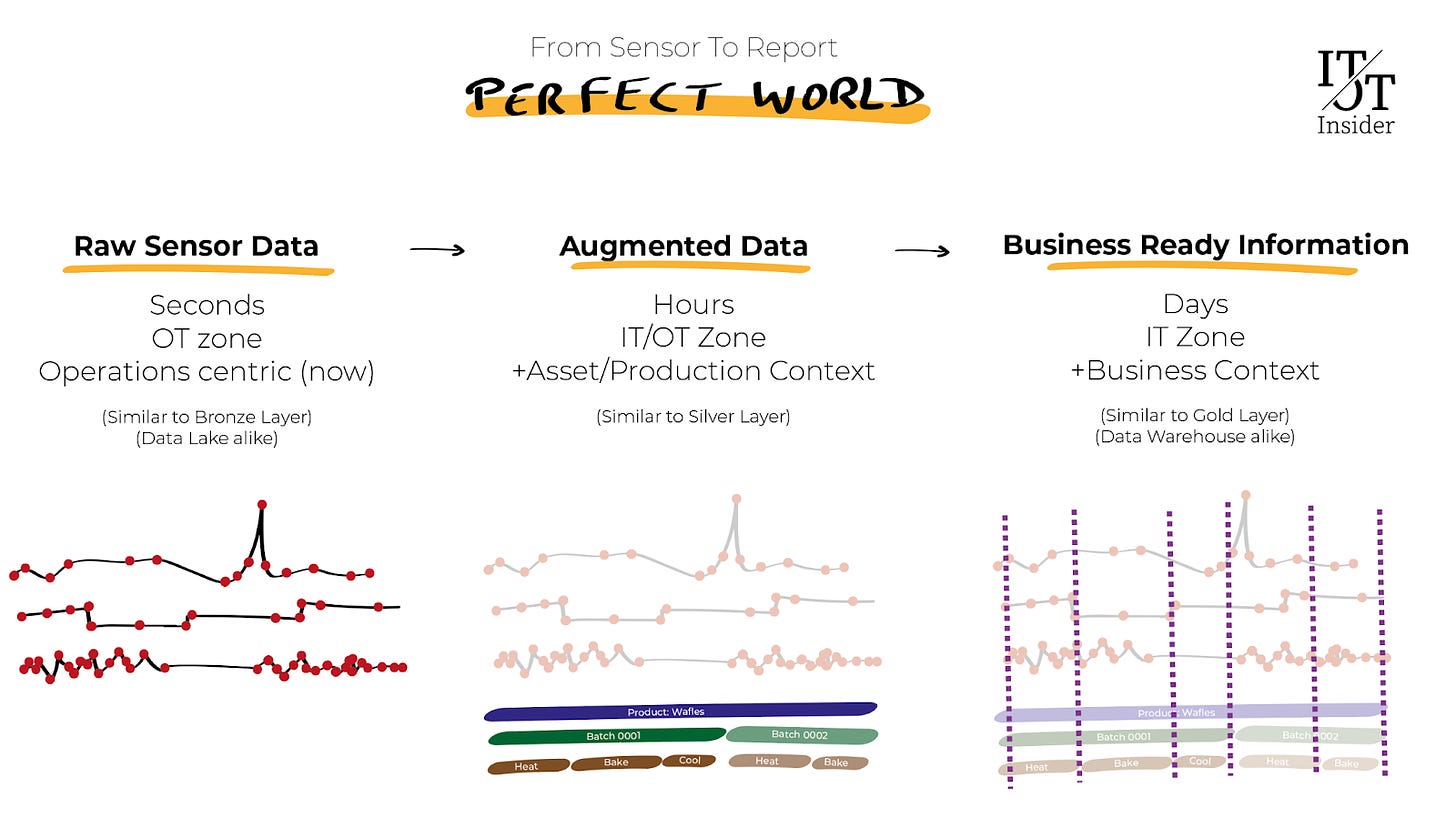
The OT view on Data Quality
First up: the OT view on Data Quality!
Let’s go back to Part 1’s cookie factory - Sweet Harmony Treats.
Sweet Harmony Treats, a name synonymous with delicious confectionary delights, was on the brink of a transformative journey. The CEO, John Dough, had a vision – a vision to enhance productivity and spark innovation within the company. His plan hinged on leveraging the vast amounts of data that were being captured daily. From the engineers to the operators on the factory floor, John envisioned a scenario where everyone could utilize this data to optimize production and streamline the supply chain. The stakes were high, but the potential rewards were even higher.
In the bustling operations room, the scene was a hive of activity. Operators and Process engineers, the guardians of the day-to-day processes, were keenly focused on their control systems. Their decisions, based on this data, were crucial in keeping the production lines humming. However, lurking beneath the surface was the ever-present threat of data quality issues. A recent incident had highlighted this vulnerability – a performance engineer, while calculating important production figures, had overlooked outliers in the data. The result was a misjudgment in production planning, causing a temporary but costly hiccup in the supply chain.
Not far away from the operators, in a quieter corner of the facility, OT engineers were deeply engrossed in their monitors, diligently monitoring the health of the sensors and automation systems. Their role was becoming increasingly complex as the data journeyed through different layers of the tech stack. They recalled an instance where an incorrect setting for a sensor post-maintenance had gone unnoticed, leading to oscillating control loops and a dip in product quality. They understood that proactive management of data quality was not just a technical requirement but a cornerstone of operational efficiency.
This should sound familiar to you if you work in OT.
Data quality has recently surfaced as a primary concern for companies that are on a digital transformation journey (based on our own experience, but also confirmed in multiple surveys).
In order to move from pilot projects to full-scale implementations of data-driven initiatives such as predictive maintenance or digital twins, a more proactive approach to managing data quality is a prerequisite.
The realization is growing that data is a strategic asset and it is about time we start treating it that way.
Even more important: as exchanging data between OT and IT systems is the main driver for IT/OT Convergence, data quality should be considered as a key success factor when setting up your Operational Data Platform.
But here’s the catch: most people in OT are not really bothered by data issues.
That is because for day-to-day operations, sensor issues are typically handled by introducing operator alerts, redundant sensors and a lot of custom scripting. Although safety issues can occur in some rare cases, production typically keeps on running if less critical sensors are out-of-service. Bypassing sensors (either via software or physically on the I/O interface) is common practice in OT.
When we start talking about Data Quality to OT people, we often hear that this is “not on their priority list” or that “people are not complaining, so why should I”. Although that might seem to be a good enough reason, it has consequences on the other side of the IT/OT fence.
The consequences on the IT side
Data good enough for Operations isn’t per se good enough for Analytics!
To make this statement tangible, let’s take a look at the data scientists and engineers working for Sweet Harmony Treats:
In the data science department, a group of AI builders faced their own set of challenges. Here, the battle was not just against the machines but also against the data itself. They spent a substantial portion of their time cleaning and preprocessing data, often grappling with bad metadata and inconsistencies across different data sets. One of the data scientists got fed up and shouted: “We don’t need better models, we need better data!”. A recent example was the optimization model that provided incorrect setpoints due to unnoticed sensor drifts. The team realized the dire need for better data context and quality to ensure their models were reliable and effective.
Meanwhile, the data engineers, the architects who crafted the data pipelines, juggled a delicate balance. They had to decide whether to duplicate data from source systems to the data platform or point directly to the source, each choice with its own set of challenges. Their decisions had direct implications on the reliability and completeness of the data being used across the company.
Observing all these dynamics from a higher vantage point was the Chief Data Officer. This role, encompassing a bird's-eye view of the entire data landscape, was critical in steering Sweet Harmony Treats’ digital transformation. The CDO’s priority list was extensive, ranging from leveraging AI/ML evolutions to ensuring data reliability and governance. A key challenge was making the hidden cost of unmanaged data quality visible and manageable. This included tackling the significant time lost in cleaning dirty data for analytics tasks and ensuring that data governance initiatives were extended to encompass OT/IoT data effectively.
As John Dough walked through the different departments, observing the concerted efforts to harness data for Sweet Harmony Treats' growth, he understood that the journey ahead was complex. Yet, he was confident that with the collective expertise and commitment of his team, the company was well on its way to transforming the challenges of today into the successes of tomorrow.
Data issues, somewhere in the pipeline from sensor to final report, are difficult to detect and correct.
You might just sum up data containing an outlier, resulting in totally wrong conclusions (1000000 instead of 1000). Or a sensor might have been given a flatline reading for days, weeks or even months without your knowledge.
When we run automatic reports or use data as part of (advanced) mathematical models (think: AI, Digital Twins…), we want to make sure we can rely on the outcome.
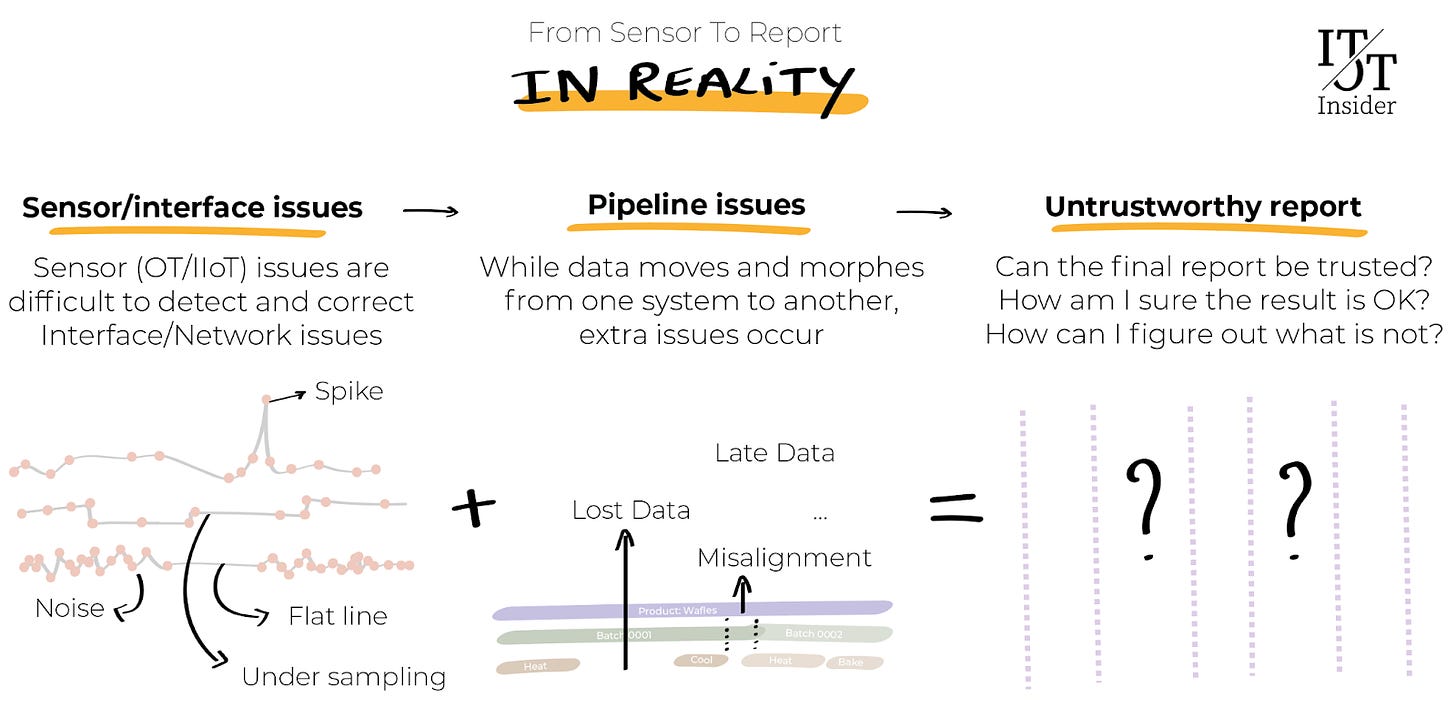
Conclusion
The hidden cost of unmanaged data quality is enormous. For instance, Forrester estimates that data teams spend 30-40% of their time handling data quality issues (from our own experience, we believe that that is an underestimation!).
With growing volumes and more use cases built on OT / IoT data, managing of these data becomes a crucial part of the digital transformation. Yet, a lot of OT / IoT data is currently unverified and unmonitored.
OT / IoT data is a strategic asset, and we should be treating it this way. Or as our Data Scientist from Sweet Harmony Treats stated it (actually that’s a quote by Andrew Ng):
We don’t need better models, we need better data!
Did this article trigger you to know more about Data Quality? Make sure to subscribe to receive our next articles!
Acknowledgment
A big thank you to Thomas Dhollander (Timeseer.AI’s CTO) for his amazing input into this article! Check out his Medium article on Data Quality as well.