It's the Platform, Stupid! Breaking the OT Data Barrier - Part 4 on Industrial Data Platforms
Explore the scaling power of data platforms in this Fourth Part on Industrial Data Platforms. Learn how achieving exponential growth is possible by breaking the data barrier with a unified approach
This is part 4 in our series on Industrial Data Platforms! In this part we are exploring why a data platform is essential. We’ll talk about scaling, faster time to value and ROI. In Part 5 we’ll discover how the Unified Namespace fits into this.
Discover all parts: Part 1 (The IT and OT view on Data), Part 2 (Introducing the Operational Data Platform), Part 3 (The need for Better Data), Part 4 (this one), Part 5 (The Unified Namespace). We also published our Data Platform Capability map, with even more insights.
Why a Data Platform is essential for any Digital Transformation plan you might have
Throwback to our article on “How the future of OT Data will look like”: most systems holding data are still on an island somewhere. That means that users and applications need to create point-to-point connections to many different systems in order to get the data they need. That slows down each and every data driven project you embark on.
Or as we described it in “The Great Unlock”:
Why is predictive maintenance so hard? Because maintenance technicians don’t want to spend hours digging through Excel sheets. When the buzzword was “Augmented Reality”, we were not looking for new ways to draw something in 3D (the gaming industry is way more advanced than the stuff we were building). No, we needed to integrate several data sources into one application and that’s exactly what was holding us back. Same now for Artificial Intelligence: the algorithms are not the problem, the data is.
IT/OT Convergence ➡️ Data Convergence ➡️ Scale Data Driven Culture
So what is the problem if we cannot scale?
Every data project becomes a massive undertaking because you need to untangle the data spaghetti over and over again!
To make matters worse, on average 70% of these projects will fail or be stopped (hopefully early enough), and for the 30% that succeed, learnings and results will typically not be shared outside of the project scope. Finally, extracting, transforming and loading data into whatever system you are using requires very specific data engineering skills making the data engineers a bottleneck for every report/trend/calculation being requested.
It’s fair to say that this way of scaling is suboptimal at its best. We typically see that with increased budgets and people, outcomes of these data-driven use cases scale less than linear !
But…
What if we would have a Data Platform integrating all OT/IIoT and maybe even IT sources with their context? Allowing us to scale the outcome of our data initiatives at least better than linear or even exponential ?
Instead of reinventing the wheel for every data use case, we should first invest in an Operational Data Platform. This data platform is managed by a platform team. Their task is to develop and maintain the platform, ensuring it meets the business’s needs. This is a product with a service model. The team operates autonomously, but they must service their users. They have full ownership of building, running, and maintaining the platform. The internal services they offer simplify life for the rest of the organization.
They need to ensure that the platform provides good quality data in context, and easy access for all types of business users (management, data scientists, reliability engineers, third parties…). They lead the governance activities but are never the data owners—that responsibility lies with the business.
The role of the platform team is to facilitate the organization in achieving its goals through a robust, scalable, and reliable data platform.
(If you like to read more about team structures - including platform teams - then you need to read the book Team Topologies)
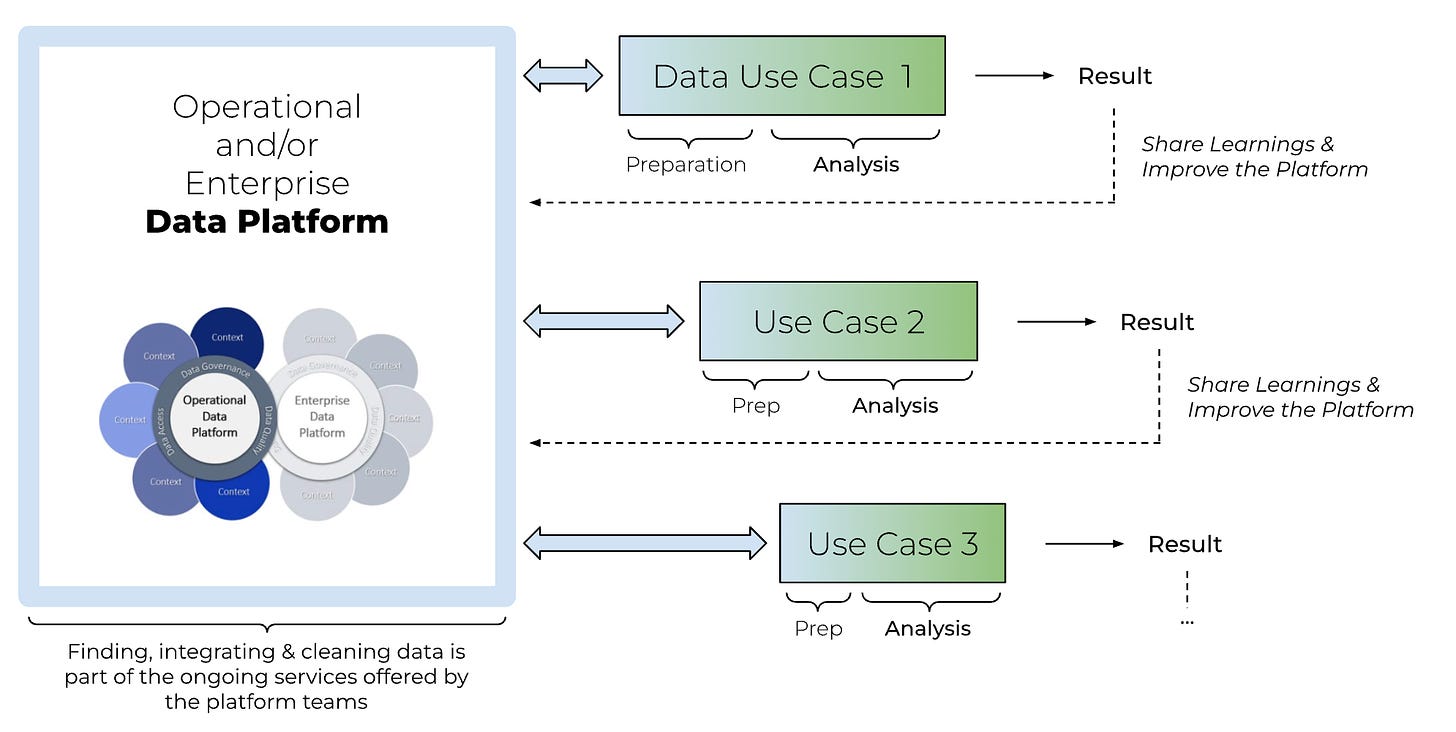
Some observations:
Centralized Data Handling:
The burden of finding, integrating, and contextualizing data is done once. A platform team takes the responsibility of providing a scalable and reliable data platform available for everyone.Incremental Implementation:
The Data Platform is not introduced as a “big bang.” Instead, a scalable foundation is laid out, and with every data use case implemented, the platform is extended, and new contexts (e.g., asset models) are added.Reduced Implementation Time:
The implementation time of a Data Use Case is drastically reduced because the big and complex task of finding, integrating, and cleaning data is now done centrally, resulting in significantly lower project risk.Shared Insights:
Results (e.g., the outcome of a machine learning model) can be easily fed back into the data platform, making these insights available to everyone. Each use case positively impacts the data set by potentially including additional cleaned data or new contextual insights.Continuous Improvement:
The implementation time of future Data Use Cases keeps decreasing as more good, contextualized and potentially cleaned data becomes available for everyone to use.
For all the reasons mentioned above, central data platforms teams have long become the status quo in the IT-world. In OT, we don’t need to reinvent the wheel 🙂
Thus…
✅ Faster Time to Value
✅ Reduced Risk
✅ Build up knowledge
… What are you waiting for 😁
Heads up: ROI
Investing in a data platform presents a challenge in justifying the Return On Investment (ROI) because the benefits are primarily indirect. Enabling technology often does not yield immediate direct returns, which can make the initial costs and efforts appear daunting. Therefore, gaining management commitment is crucial. Leadership must understand and buy into the bigger picture, recognizing that the true value of a data platform lies in the long-term advantages it provides.
As we have shown in the People/Budget/Time vs Outcomes graph, the actual savings and returns come from decreased project risk, faster turnaround times, and the systematic capture and reuse of knowledge. Achieve more, with less people, against less budget.
A centralized data platform reduces the risk associated with data integration and management, shortens the time needed to implement new projects and make data-driven decisions, and ensures valuable insights are retained across different initiatives. Additionally, the platform enhances scalability, flexibility, decision-making, and collaboration across departments, ultimately leading to significant long-term savings and operational efficiencies. Management's commitment to this vision is essential for realizing these benefits.
Sounds amazing! How do I get started?
There are some challenges to overcome!
Secure and Scalable Connectivity:
We need a secure and scalable connectivity layer to integrate different data sources into one platform (taking into account that we need to connect to old assets using legacy protocols as well as new assets equipped with the latest shiny bells and whistles);Data Models Management:
We need to add and govern different data models;Data Storage Solutions:
We need a way to store the resulting data sets (both the raw data, but also prepared and cleaned data sets) in a platform (edge, cloud or hybrid) which can handle sensor data at scale.
These requirements can easily be mapped onto our Operational Data Platform vision:
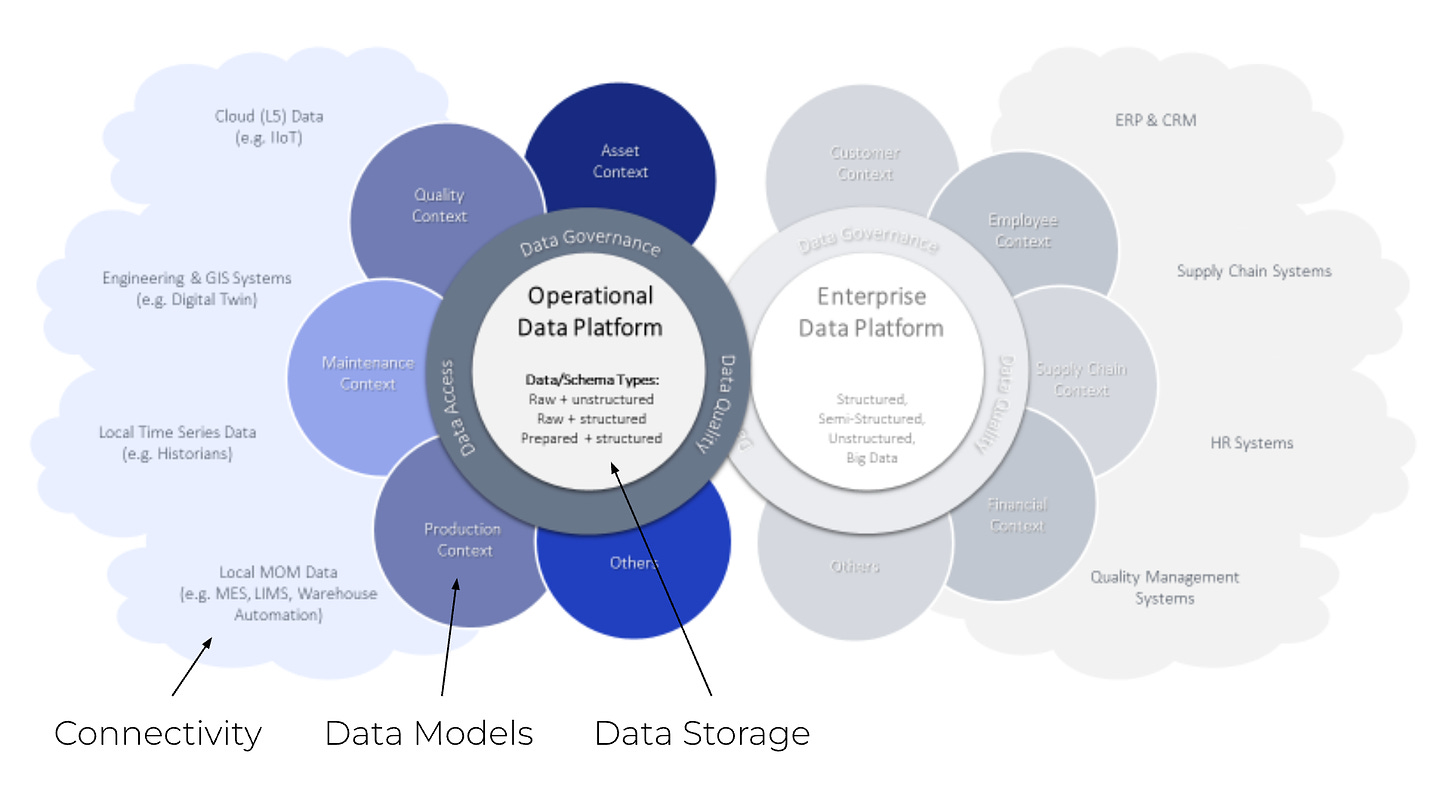
In Part 5 article we will introduce the Unified Namespace (UNS) as a possible design pattern to make this vision a reality !
As a teaser, here is our definition of a UNS:
A Unified Namespace is
an organized and centralized data broker,
depicting data in context from your entire business
as it is right now.
Discover all parts
Part 1 (The IT and OT view on Data), Part 2 (Introducing the Operational Data Platform), Part 3 (The need for Better Data), Part 4 (Breaking the OT Data Barrier: It's the Platform), Part 5 (The Unified Namespace)
Acknowledgment
Special thanks to Ivo Everts, Sr. Strategic Architect at Databricks for his time and valuable input.
We are looking for your data platform story!
🎉 Do you have a Data Platform story to share? We would love to have you on the podcast or (if you prefer written only) write down your case study! Let me know via david@itotinsider.com
Looking for an image to share?
(please, credit The IT/OT Insider)
Why 2 data platforms (operational and enterprise), instead of just one ? Modern stacks like Databricks or MS Fabric can handle it in my opinion. It seems to me that the key is to have one centralized platform for both OT and IT data and a strong collaboration between OT and IT professionals around de governance of it. I’m really interrested to better your vision.